V Ya
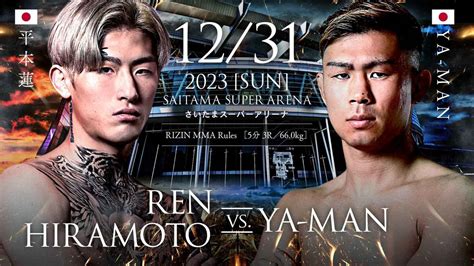
Artificial intelligence (AI) has revolutionized numerous aspects of our lives, from how we interact with technology to how businesses operate. One of the most significant impacts of AI has been in the field of natural language processing (NLP), enabling computers to understand, interpret, and generate human language. This advancement has led to the development of chatbots, virtual assistants, and language translation software, among other applications. The integration of AI in NLP has not only improved the efficiency and accuracy of language-related tasks but has also opened up new avenues for research and innovation.
Key Points
- The evolution of AI in NLP has transformed how humans interact with machines, making it more intuitive and user-friendly.
- Applications of AI in NLP include chatbots, virtual assistants, sentiment analysis, and language translation software.
- The future of NLP is anticipated to involve more sophisticated models that can understand nuances of human language, including sarcasm, irony, and context-specific expressions.
- Challenges facing the development of NLP include handling ambiguities in language, dealing with the vastness of human vocabulary, and ensuring cultural sensitivity.
- The economic impact of NLP is significant, with applications in customer service, content generation, and data analysis, potentially saving businesses millions of dollars annually.
Natural Language Processing: A Fundamental Component of AI

NLP is a subfield of AI that focuses on the interaction between computers and humans in natural language. It combines computer science, artificial intelligence, and linguistics to enable computers to process, understand, and generate natural language data. The development of NLP has been gradual, with early systems relying on rule-based approaches to more recent models that utilize machine learning and deep learning techniques. These advancements have significantly improved the accuracy and efficiency of NLP systems, allowing them to be applied in a wide range of domains.
Machine Learning and Deep Learning in NLP
The integration of machine learning and deep learning in NLP has been a game-changer. Machine learning algorithms enable NLP systems to learn from data, improving their performance over time. Deep learning models, such as recurrent neural networks (RNNs) and transformers, have further enhanced the capabilities of NLP systems by allowing them to capture complex patterns and relationships in language. These models have been instrumental in achieving state-of-the-art results in various NLP tasks, including language translation, question answering, and text summarization.
NLP Task | Machine Learning Approach | Deep Learning Approach |
---|---|---|
Language Translation | Statistical Machine Translation | Neural Machine Translation |
Text Classification | Naive Bayes, SVM | Convolutional Neural Networks (CNN), Recurrent Neural Networks (RNN) |
Sentiment Analysis | Rule-Based Systems | Long Short-Term Memory (LSTM) Networks, Transformers |

Applications of NLP

NLP has a wide range of applications across various industries. In customer service, chatbots powered by NLP can provide 24⁄7 support, answering frequent queries and helping to resolve issues. Virtual assistants like Siri, Alexa, and Google Assistant rely on NLP to understand voice commands and perform tasks. Language translation software has made it possible for people who speak different languages to communicate effectively, bridging cultural gaps. Furthermore, NLP is used in sentiment analysis to gauge public opinion on products, services, and social issues, providing valuable insights for businesses and policymakers.
Challenges in NLP
Despite the significant progress made in NLP, there are several challenges that researchers and developers face. One of the major challenges is handling the ambiguity and complexity of human language. Words can have multiple meanings, and the same sentence can be interpreted in different ways depending on the context. Another challenge is dealing with the vastness of human vocabulary and the constant evolution of language, with new words and expressions being added all the time. Ensuring that NLP systems are culturally sensitive and can understand nuances like sarcasm, irony, and figurative language is also a significant challenge.
What are the primary applications of NLP in business?
+The primary applications of NLP in business include customer service chatbots, virtual assistants, sentiment analysis for market research, and automated content generation. These applications can significantly enhance customer experience, improve operational efficiency, and provide valuable market insights.
How does NLP contribute to language translation?
+NLP contributes to language translation by enabling computers to understand and interpret the nuances of language, including grammar, syntax, and semantics. This allows for more accurate and natural-sounding translations, facilitating communication across language barriers.
What are the future directions of NLP research?
+Future directions of NLP research include the development of more sophisticated models that can understand the context and nuances of human language, the integration of multimodal processing to handle different forms of data, and the application of NLP in emerging areas like healthcare and education.
In conclusion, the evolution of AI in NLP has transformed the way humans interact with technology, enabling more intuitive, efficient, and accurate communication. As NLP continues to advance, we can expect to see more sophisticated applications that not only understand the complexities of human language but also generate human-like responses. The future of NLP holds much promise, with potential applications in virtually every domain of human activity, from enhancing customer service and improving language translation to facilitating multicultural communication and understanding.